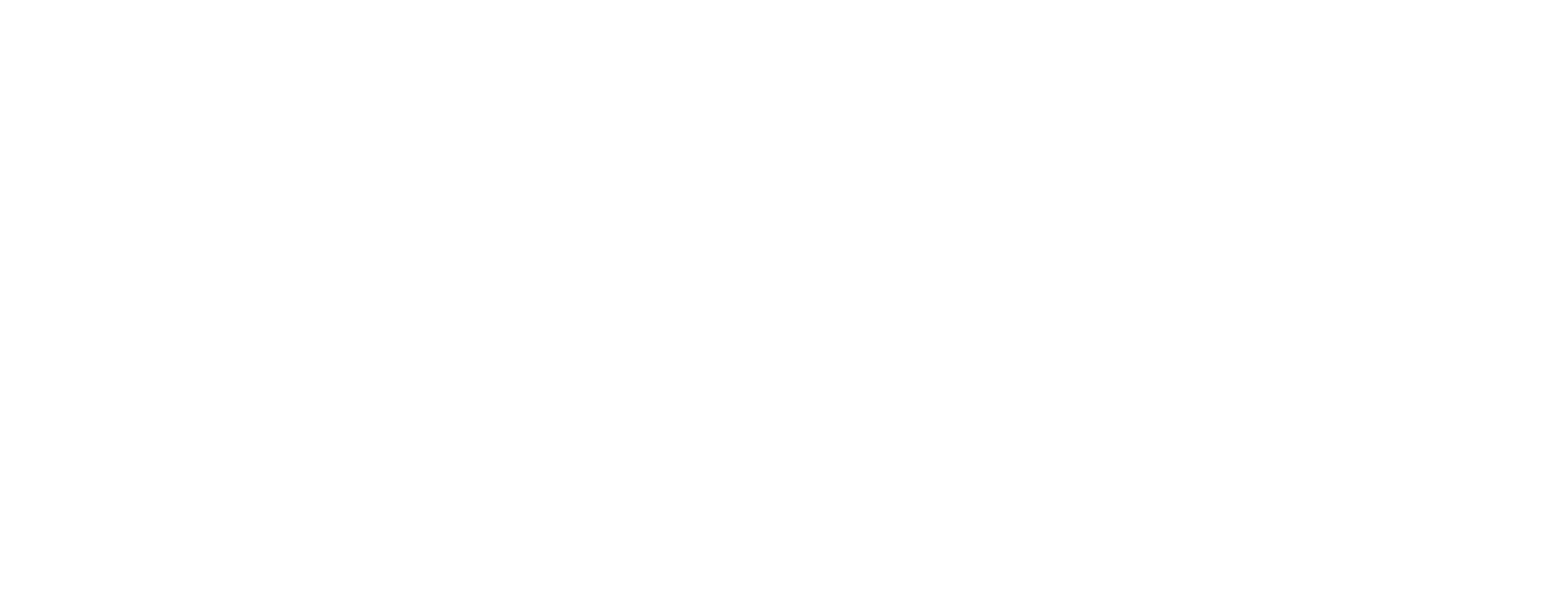
About
SpaceLab: Data Science for Space Research is a working group within the Department of Knowledge Technologies at the Jožef Stefan Institute. It facilitates the data-to-discovery process in space research by applying AI methods across the complete data life cycle. The data life cycle includes data storage, data stewardship, data and knowledge representation, machine learning/ analysis and visualization. Each of these stages needs to be equipped with AI methods: starting from semantic representations and stores of data, models and knowledge, through explainable AI methods to successful discovery and decisions.
SpaceLab deals with analysis of large amounts of scientific and complex data collected through space missions, as well as data about the operations of such missions. Scientific data are collected by Earth Observation missions or observation of other planets in our solar system or even observations of distant parts of the universe (e.g., collecting various spectra for different celestial bodies in astrophysics). Added value can be derived by analyzing such data for a variety of scientific and practical applications, but the complexity and sheer volume of such data present numerous obstacles. In addition, added value can also be derived by re-using the collected data by different actors from industry or the public sector, but this is impeded, among other things, by the lack of appropriate annotations of such data and consequent difficulty of its discoverability. Increasingly large amounts of operations data are collected for the many current space missions, which are typically only used by experts operating the spacecraft manually, to monitor the spacecraft operations. Added value can be derived by analyzing such data and building predictive models, which can, for example, predict electrical power consumption and facilitate planning for better utilization of the spacecraft. Furthermore, many space missions use similar spacecraft, so data science workflows developed for one spacecraft can be readily re-used, as long as they are formalized through ontological annotation.
SpaceLab focuses on design, development and deployment of advanced AI solutions for modelling complex and dynamic solutions. We adhere to the principles of transparent AI: the semantic representation enables traceability of both data and models, while the methods we develop provide means to explainability and understandability of the models by domain experts.
-
Dragi Kocev (scientific lead)
-
Panče Panov
-
Matej Petković
-
Ana Kostovska
-
Tomaž Stepišnik
-
Nikola Simidjievski
Team
Projects
Machine learning for predicting thermal power consumption of the Mars Express Spacecraft
The thermal subsystem of the Mars Express (MEX) spacecraft keeps the on-board equipment within its pre-defined operating temperatures range. To plan and optimize the scientific operations of MEX, its operators need to estimate in advance, as accurately as possible, the power consumption of the thermal subsystem. The remaining power can then be allocated for scientific purposes.
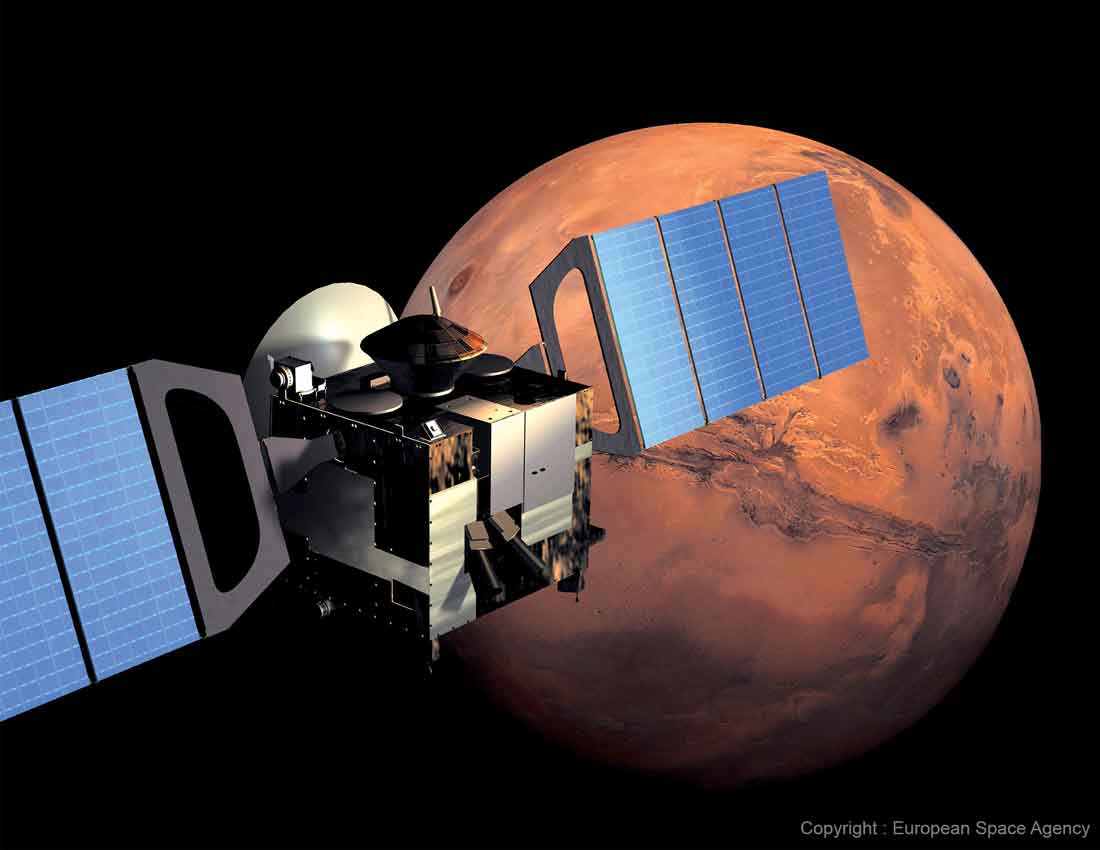
Quantifying the effects of gyroless flying of the Mars Express Spacecraft
The gyroscopes on-board the Mars Express (MEX) spacecraft, responsible for orientation and pointing actions, are slowly decaying. On 16th April 2018, a new software was deployed to MEX which reduces gyroscope usage by 90%. We investigate the effect of gyroless flight on the power consumption of MEX’s thermal subsystems.
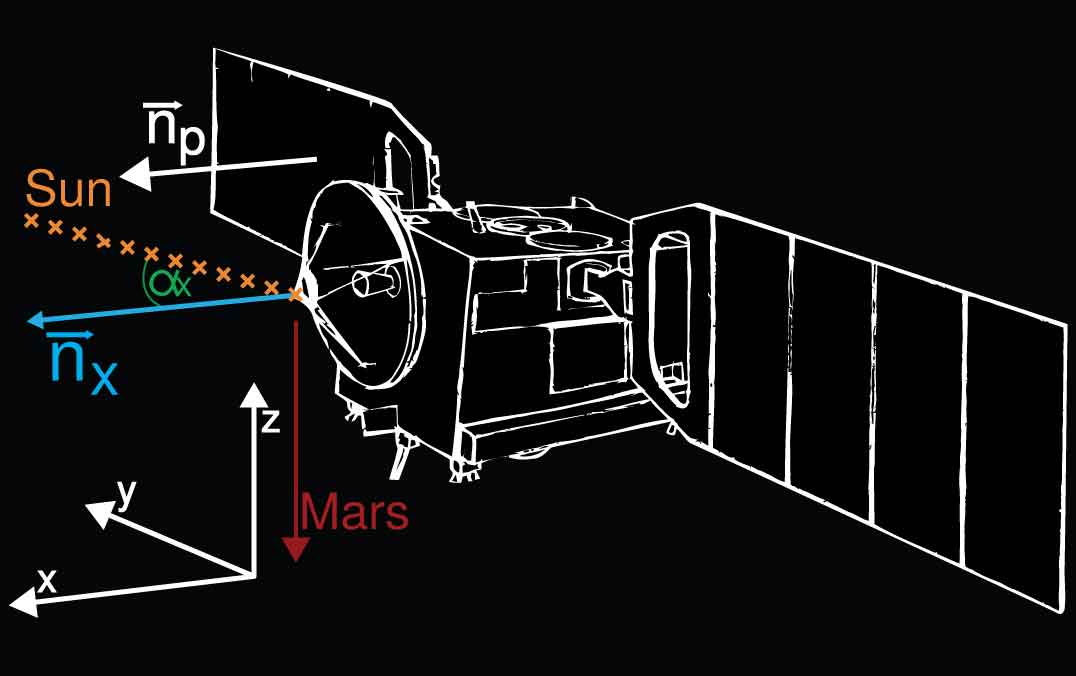
In the media
- (2016) Winning team of ESA s Mars-focused data_mining contest - European Space Agency Official
- (2016) TV interview for the Slovenian national public television RTVSLO (in Slovene)
- (2016) Radio interview for the Slovenian national public radtio Radio1 (in Slovene)
- (2016) Article in the biggest Slovenian newspaper Delo (in Slovene)
- (2016) Article by the Slovenian Natioanl Press Agency (in Slovene)